Aging Infrastructure, New Tools: The RFPhantom Cable Modem Poller
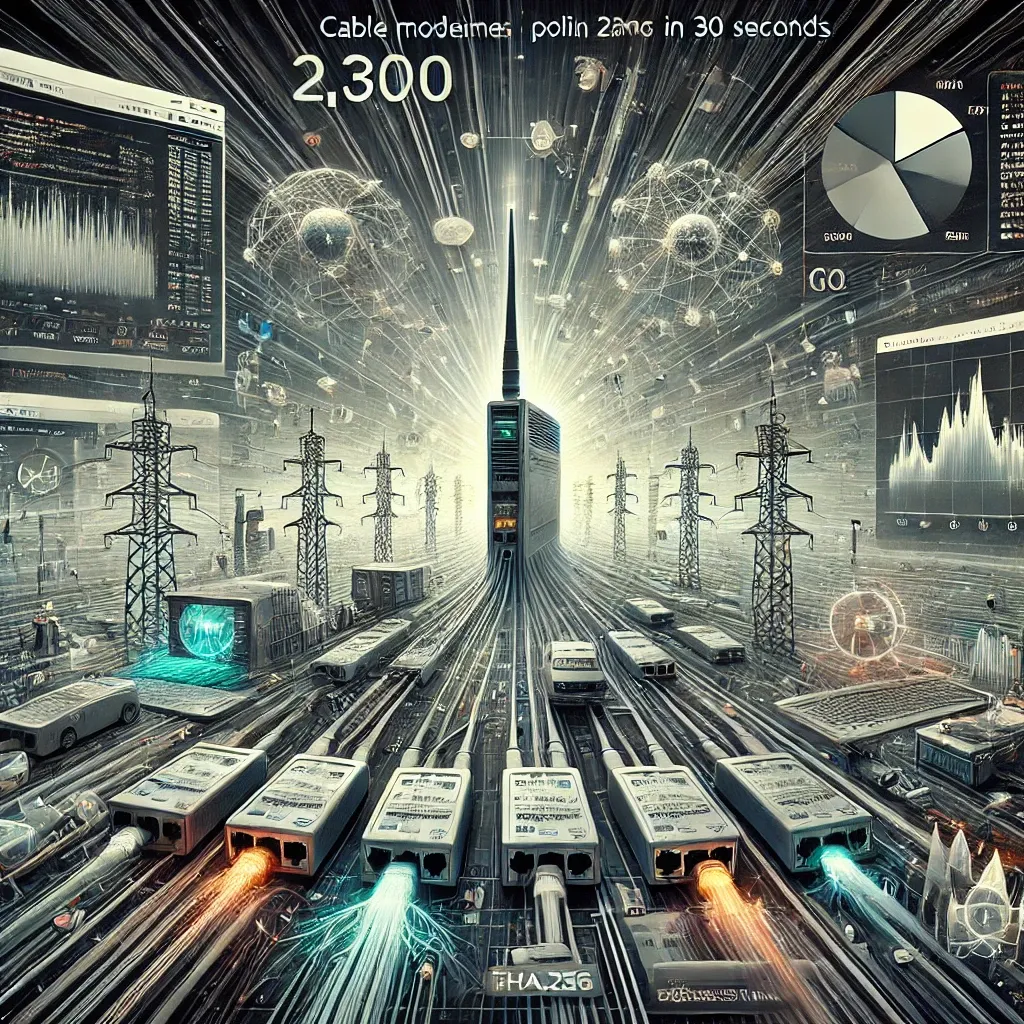
A Brief (Well, Not So Brief) Introduction
What started as a simple blog post to share a few insights quickly snowballed into something much more—well, let’s just say it turned into a short book. It seems that once you start unraveling the complexities of network monitoring and modem polling, it’s hard to stop! So buckle up for a deep dive that began with modest intentions but evolved into a comprehensive guide on everything from the basics of cable modems to the intricacies of data ingestion. Here’s hoping you find as much value in reading it as I found in writing it!
Why This Matters: The Persistence of Legacy Tech
Sure, fiber is the future, and it's gaining ground fast. But let's be honest—there are still plenty of places where legacy technologies like Cable Modems and DOCSIS are going to be sticking around for years, maybe even decades. These systems have been the backbone of connectivity in countless communities since the late 1990s, when cable modems first started rolling out as a faster, more reliable alternative to dial-up. They represented a massive leap forward, bringing broadband to millions of households and laying the groundwork for the internet as we know it today.
But time marches on, and while the technology might be old by today’s standards, the demand for reliable, high-performance tools hasn’t diminished. In fact, as this infrastructure ages, the need for robust monitoring and maintenance tools is more crucial than ever. Aging cables, old amplifiers, and the wear and tear of decades of use mean that keeping these systems running smoothly is a growing challenge.
That’s where this project comes in. While the world keeps pushing forward, there’s a real need to keep these older systems not just operational, but optimized. We need new tools to handle the quirks, challenges, and demands of these aging networks—tools that can ensure these legacy systems continue to deliver the reliability and performance that people have come to depend on. So, if you’re in a place where fiber isn’t quite ready to take over, you’ll appreciate why projects like this one are still so vital.
A Brief History of Cable Modems and DOCSIS
The story of cable modems and DOCSIS (Data Over Cable Service Interface Specification) begins in the mid-1990s, at a time when the internet was still in its infancy for most households. Back then, dial-up connections were the norm, and while they served their purpose, they were painfully slow by today’s standards. The need for faster, more reliable internet access was becoming increasingly apparent as the web expanded and more data-intensive applications began to emerge.
The Early Days: Laying the Groundwork
Cable television companies, already established with vast networks of coaxial cables running into millions of homes, saw an opportunity. These networks, originally designed to deliver television signals, had untapped potential for carrying data. Engineers and innovators began experimenting with ways to transmit digital data alongside television signals, leading to the development of the first cable modems.
These early cable modems were a game-changer. Unlike dial-up, which required a dedicated phone line and offered limited bandwidth, cable modems could provide always-on internet access with significantly higher speeds. The technology was quickly embraced, and by the late 1990s, cable internet was rolling out in cities across the United States and around the world.
The Birth of DOCSIS
With the rapid adoption of cable internet came the need for standardization. In 1997, the first version of DOCSIS was released. DOCSIS provided a standardized way for cable operators to offer broadband internet over their existing cable television systems. It specified how data should be transmitted over the cable network, including everything from modulation techniques to security protocols.
DOCSIS was revolutionary for several reasons:
- Interoperability: By providing a standard, DOCSIS allowed different manufacturers to produce cable modems and headend equipment that could work together seamlessly. This was crucial for the widespread adoption of cable internet.
- Scalability: DOCSIS was designed to be scalable, allowing operators to upgrade their networks and offer faster speeds as technology advanced.
- Quality of Service (QoS): DOCSIS included provisions for prioritizing different types of traffic, ensuring that critical data (like voice over IP) could be delivered reliably even as network congestion increased.
Evolution and Advancements
Since its initial release, DOCSIS has undergone several major revisions, each one bringing enhancements that allowed cable operators to offer faster speeds and more reliable service:
- DOCSIS 1.0 (1997): The first standard, allowing speeds up to 40 Mbps downstream and 10 Mbps upstream, though in practice, speeds were typically much lower.
- DOCSIS 2.0 (2001): Introduced improvements to upstream speeds, a critical enhancement as the demand for more interactive services (like file sharing and VoIP) grew.
- DOCSIS 3.0 (2006): A significant leap forward, introducing channel bonding, which allowed multiple channels to be combined to increase data throughput. This version made it possible for operators to offer speeds well over 100 Mbps.
- DOCSIS 3.1 (2013): This version introduced support for gigabit speeds, using more advanced modulation techniques and further enhancing network efficiency. It also included improvements to latency and support for IPv6.
- DOCSIS 4.0 (2020): The latest version, which pushes the technology even further, supporting multi-gigabit speeds both downstream and upstream. It also enhances network security and prepares cable networks for the increasing demands of the future.
The Present and Future
Today, cable modems and DOCSIS continue to play a crucial role in global internet infrastructure. Even as fiber optic networks expand, cable remains a dominant force, particularly in regions where laying new fiber is cost-prohibitive or logistically challenging. DOCSIS technology has allowed cable operators to keep pace with the increasing demand for higher bandwidth, enabling millions of households to access high-speed internet.
However, as with any aging technology, the infrastructure supporting cable internet is starting to show its age. Coaxial cables, amplifiers, and other network components that have been in service for decades require more maintenance and monitoring. This is where modern tools and systems, like the modem poller project, become indispensable. They help operators manage and optimize these aging networks, ensuring that customers continue to receive reliable service even as the industry gradually transitions to newer technologies.
In summary, while cable modems and DOCSIS might seem like relics of a bygone era to some, they are still very much relevant and vital in today’s internet landscape. Their evolution over the past few decades is a testament to the adaptability of the technology and its ability to meet the ever-growing demands of digital communication.
Old Tech, New Tools: The Game Never Ends
As we’ve seen, cable modems and DOCSIS have been the workhorses of broadband internet for decades, and while the infrastructure may be aging, the demand for reliable connectivity hasn't wavered. The cables laid down years ago are still humming along, carrying data to millions of households every day. But as any network engineer will tell you, time is not kind to technology. Coaxial cables degrade, amplifiers wear out, and network noise becomes a bigger issue as more devices compete for bandwidth.
This aging infrastructure presents unique challenges. The technology might be old, but the problems it faces are very much of the present. Latency spikes, signal degradation, and bandwidth bottlenecks are just a few of the issues that can crop up in a network that’s been in service for decades. As the network ages, these problems become more frequent and harder to diagnose. The need for sophisticated tools to monitor, diagnose, and maintain these networks has never been greater.
Enter the idea of "Old Tech, New Tools." Just because the infrastructure is old doesn't mean the tools we use to manage it should be. In fact, it’s the opposite. The older the network, the more it benefits from modern monitoring and maintenance solutions. The game never ends when it comes to keeping these networks running smoothly. Network operators must constantly innovate, finding new ways to squeeze more performance and reliability out of aging hardware.
This is where the RFPhantom project comes in. It’s a modern solution designed to tackle the very real challenges of maintaining legacy networks. By leveraging the latest in data collection, processing, and analysis, RFPhantom breathes new life into aging infrastructure. It offers the tools necessary to monitor network health in real-time, diagnose issues before they become critical, and ensure that even the oldest networks can keep up with the demands of today’s internet users.
RFPhantom isn't just about keeping old systems running—it's about optimizing them, pushing them to deliver the best possible performance even as they age. In a world where the next big thing is always on the horizon, projects like RFPhantom remind us that sometimes the best way forward is to make the most of what we already have, with tools that are as modern as the challenges they address.
Revisiting the Old Tool: A Decade in the Making
I’ve written about my old tool in previous blog posts, but let’s rehash it briefly here for context. Nearly 15 years ago, I built a solution using the LAMPP stack—LAMP for storage and the front end, and Perl handling the backend. It was cutting-edge for its time, built to manage and monitor cable modem networks when DOCSIS was still making waves.
But as I discuss deeply in this post From Perl to Go the limitations of that stack became apparent as technology advanced. The LAMPP stack was powerful, but it was constrained by the processing and memory capabilities of the time. Perl served me well, but as the network demands grew, it struggled to keep up with the need for more efficient data handling and faster processing.
Fast forward to today, and we have new tools at our disposal. Platforms for data analytics have evolved, offering real-time insights and the ability to handle vast amounts of data with ease. Languages like Go have emerged, providing the simplicity of Perl but with far superior memory management and threading capabilities. This shift has enabled us to build systems that are not only faster but also more resilient and scalable.
The RFPhantom project is the evolution of that old tool—rebuilt from the ground up with modern technology to meet the needs of today’s networks. We’re not just keeping up with the times; we’re staying ahead of them.
How Cable Modems Really Work
Cable modems are often thought of as straightforward devices—just plug them in, and they magically connect you to the internet. But the reality is a bit more complex, especially when you consider the journey your data takes. Unlike fiber connections, which use light to transmit data end-to-end, cable modems rely on a mix of analog and digital signals.
Here’s a simplified rundown:
- Analog to Digital Conversion: Your cable modem receives an analog signal from the coaxial cable running into your home. This analog signal carries data, much like it carries TV channels, but instead of just displaying content, the modem’s job is to convert this analog signal into a digital one that your computer can understand.
- Transmission to the CMTS: Once converted to digital, your data is sent upstream to a Cable Modem Termination System (CMTS) at your ISP’s headend. This part of the journey is all digital, but it’s happening over infrastructure that was originally designed for analog TV, not high-speed internet.
- Analog to Light and Back: Here’s where it gets interesting. Before reaching the CMTS, your digital data is often converted back light and then transmitted over fiber optics. But it’s not just the data that gets converted—any noise present in the analog signal is also transmitted along with the light. When the signal reaches the coaxial network at the ISP, it’s converted back to analog. The real cleanup of this noise is done at the modem and the CMTS, the analog parts of the system.
Because of this complex signal journey, every metric you can track becomes critical. The analog nature of parts of the system means that noise, signal strength, and timing can all have a significant impact on your connection quality. A small issue, like a degraded signal due to a worn-out cable or a poorly aligned amplifier, can cause significant problems.
This is why it’s so important to monitor every feasible metric without overloading the system. You need to keep an eye on signal-to-noise ratios, upstream and downstream power levels, latency, and error rates—all of which can give you early warning signs of potential issues. By tracking these metrics, you can maintain the health of the network, troubleshoot problems quickly, and ensure that the analog-to-digital dance happens smoothly, keeping everyone connected.
Getting to the Crux
Monitoring a cable modem is much like monitoring a router, but with a few twists. Depending on the configuration, we could be collecting data from as few as four interfaces or up to 40 interfaces in some cases. Your cable modem’s data path is broken up into a number of channels before beginning its journey, and those channels are managed by both the cable modem and the CMTS.
To get a full picture of the network’s health, we have to gather as much data from these interfaces as we can. Unlike the typical Ethernet interfaces most are used to, cable modems also have signal metrics on every RF channel. These metrics are where our story lies—capturing the nuances of signal strength, noise levels, and other critical factors that can make or break the quality of your internet connection.
In phase one of this project, we’re scanning 2,300 cable modems. Think of it as monitoring 2,300 individual routers, each with up to 40 interfaces that we need to track. What’s particularly interesting is that two cable channels on the same RF coax can show different RF metrics, even when they’re right next to each other. There are a boatload of reasons why this can happen—everything from interference to physical damage in the cable—and that’s exactly what this tool helps us figure out. By gathering and analyzing this data, we can pinpoint issues that might otherwise go unnoticed, ensuring the network runs as smoothly as possible.
What Metrics Do We Actually Collect?
In Phase 1 of this project, we're focusing on a specific set of DOCSIS metrics that give us crucial insights into the performance and reliability of cable modems. These metrics allow us to monitor the signal quality, error rates, and overall health of the modem's connection. Here’s a breakdown of the metrics we're collecting and what they reveal about the network:
1. docsIfDownChannelPower
- What It Gives Us: This metric measures the downstream power level received by the modem. It’s crucial for ensuring that the signal strength is within an optimal range. If the power level is too low, the modem might struggle to maintain a connection, while too high a level can cause signal distortion and overload the modem’s receiver.
2. docsIfSigQUnerroreds
- What It Gives Us: This metric counts the number of codewords (blocks of data) that were received without any errors. A high count of unerrored codewords indicates a clean and stable signal, which is essential for maintaining high-speed data transmission.
3. docsIfSigQCorrecteds
- What It Gives Us: This metric tracks the number of codewords that had errors but were corrected by the modem using forward error correction (FEC). While it’s good that the modem can correct these errors, a high number of correcteds may indicate that the signal quality is starting to degrade.
4. docsIfSigQUncorrectables
- What It Gives Us: This metric records the number of codewords that had errors that could not be corrected. Uncorrectable errors are a serious issue as they lead to data loss and can cause noticeable disruptions in service, such as slow speeds or dropped connections.
5. docsIfSigQSignalNoise
- What It Gives Us: This measures the signal-to-noise ratio (SNR) for the downstream signal. A high SNR is indicative of a clean signal with minimal interference, which is vital for efficient data transmission. A low SNR can result in higher error rates and reduced throughput.
6. docsIfSigQMicroreflections
- What It Gives Us: Microreflections occur when the signal is reflected back into the cable system due to impedance mismatches, creating echoes that can interfere with the main signal. This metric tracks the severity of these microreflections, which can degrade signal quality and lead to errors.
7. docsIfCmStatusT3Timeouts
- What It Gives Us: T3 timeouts occur when the modem doesn’t receive a response from the CMTS within the expected timeframe during the ranging process (where the modem adjusts its timing to sync with the CMTS). A high number of T3 timeouts can indicate upstream issues, such as noise or interference, that are preventing the modem from communicating effectively with the CMTS.
8. docsIfCmStatusT4Timeouts
- What It Gives Us: T4 timeouts are more severe than T3 timeouts and occur when the modem fails to maintain its connection with the CMTS altogether. This can lead to the modem resetting its connection, causing interruptions in service. Monitoring T4 timeouts helps us detect critical connectivity issues early.
9. docsIfCmStatusRangingAborteds
- What It Gives Us: This metric counts the number of times the ranging process was aborted. Ranging is essential for the modem to synchronize with the CMTS, and aborted attempts can indicate significant upstream issues that need to be addressed to maintain a stable connection.
10. docsIfCmStatusTxPower
- What It Gives Us: This metric measures the transmission power of the modem’s upstream signal. The modem adjusts its transmission power to ensure that its signal reaches the CMTS with the proper strength. If the Tx power is too high or too low, it could indicate problems like line attenuation or excessive noise on the upstream channel.
Why These Metrics Matter
These metrics are critical for monitoring the health and performance of the cable modems in the network. They provide detailed insights into the quality of the signal, the presence of errors, and the modem's ability to maintain a stable connection. By collecting and analyzing this data, we can proactively identify and address issues, ensuring that the network operates smoothly and that users experience reliable, high-quality service.
This is just Phase 1. We have more metrics and more math to bring into the foray in the future, but for Phase 1, these were a great place to start.
Now on to What Everyone Has Been Waiting For: How We Poll 2,300 Modems in Less Than 30 Seconds
Polling 2,300 modems is no small feat, especially when you consider the scale of data involved. If you take those 2,300 modems and multiply them by an average of 25 interfaces per modem, and then factor in an average of 10 metrics per interface—understanding that upstream and downstream channels each have different sets of values to poll—you start to see the magnitude of the challenge. That’s tens of thousands of data points to collect, process, and analyze in a very short amount of time.
And it’s not just a matter of polling the modems directly. Before we even begin querying the modems themselves, we have to poll the CMTS (Cable Modem Termination System) four times. Each of these preliminary polls is essential to gathering the context we need to make sense of the data coming from the modems. It takes some serious thought and clever engineering to wrangle all of this data efficiently without overwhelming the network or the system collecting the data.
In the sections that follow, we’ll break down how each part of this process works—from the initial CMTS polling to the final data collection from the modems. We’ll explore the techniques and strategies that allow us to form a complete, real-time picture of the network, all within a matter of seconds.
The Project Tree
Before we dive into the nuts and bolts of how we poll 2,300 modems in less than 30 seconds, let’s take a look at the project tree. This structure lays out all the components of the system, giving us a clear roadmap of how everything is organized. Each file and directory plays a crucial role in making this process work seamlessly. As we step through the polling process, we’ll reference these items to explain how they contribute to the overall operation. Here’s what the project looks like:
. ├── config │ ├── LoadCli.go │ ├── LoadConfig.go │ └── LoadEnvConfig.go ├── go.mod ├── go.sum ├── main.go ├── modempoller │ ├── BootstrapModemPollers.go │ ├── BuildOIDChunks.go │ ├── ConnectToOpensearch.go │ ├── CreateModemStateIndex.go │ ├── EnrichModemData.go │ ├── GetModemsFromCMTS.go │ ├── GetSNMPChunks.go │ ├── IngestModemDataToOpenSearch.go │ ├── ModemDataStruct.go │ ├── ProcessModemIfType.go │ ├── QueryUserInfoFromParser.go │ ├── SharedVarsAndFunctions.go │ └── StartModemPollerThreads.go └── cmts.env
It’s worth noting that this was my first large-scale Go project. As with any initial version, there’s always room for evolution and cleanup over time. But for this first version that went to staging, this setup worked. It’s a solid foundation, and I’m confident that as the project progresses, it will only get better and more refined.
main.go: The Entry Point
As the heart of the program, main.go
is the entry point that kick-starts the entire modem polling process. It’s where everything begins, laying the groundwork for the tasks that follow. Here’s how it all unfolds:
- Processing CLI Options: The first task
main.go
handles is processing the command-line interface (CLI) options. These options allow for flexible configurations when running the program, giving you control over various parameters without needing to alter the code itself. - Loading Configuration: Next, it loads the configuration file, which in this case is formatted as
.env
. The .env file can be named whatever fits your infrastructure as it is loaded from the command line option -config. This file contains key settings and parameters necessary for the program to function correctly. By loading this configuration, the program ensures it has all the necessary information before proceeding. - Checking for Modem State Index: With the configurations in place,
main.go
checks for the existence of themodem_state_index
in OpenSearch. This step is crucial because it ensures that the index, where all the modem data will be stored and queried, is available and ready. - Verifying the Connection to OpenSearch: The program then verifies its connection to OpenSearch. This step ensures that the database is accessible and that the program can send and receive data without any issues. If the connection isn’t verified, the polling process can’t proceed.
- Loading the ModemData Memory Struct: Before any polling begins,
main.go
loads theModemData
memory struct. This structure serves as a temporary storage space for the data collected during the polling process, allowing the program to efficiently process and manipulate the data before it’s sent to OpenSearch. - Passing Control to BootstrapModemPollers: Finally, with all the preliminary steps completed,
main.go
passes control to theBootstrapModemPollers
function. This is where the actual polling begins, withBootstrapModemPollers
orchestrating the collection of data from the 2,300 modems.
The modem_state_index
The modem_state_index
is a crucial part of our data architecture, but it serves a very different purpose than a traditional time series index. Unlike indices that track historical data over time, the modem_state_index
is designed to hold the latest snapshot of each modem's state. This approach allows us to maintain a clean and efficient system that can quickly provide a real-time view of the network.
What It Stores
The modem_state_index
doesn’t accumulate time series data; instead, it keeps just the most recent state of each modem. This is accomplished by generating a unique SHA-256 key for each modem that combines several critical pieces of information:
- Modem MAC Address: The unique identifier for the modem itself.
- Location: Where the modem is physically located within the network.
- Current Owner: Who currently has the modem, which can be essential for tracking changes and ownership.
This SHA-256 key acts as a unique identifier in OpenSearch, ensuring that each modem's state is updated efficiently and without duplication.
How It Works
Every time the poller runs, it updates the modem_state_index
with the latest state of each modem. The system uses the SHA-256 key as the OpenSearch ID, meaning that if the modem's state has changed since the last poll, the existing entry is updated rather than creating a new one. This keeps the index tidy and ensures that we always have the most up-to-date information available at a glance.
The Benefits
This approach provides several key advantages:
- Fast Access to Current Data: Since the
modem_state_index
only contains the latest snapshot of each modem, queries are extremely fast. This allows us to generate real-time maps and views of the network, showing the current state of modems without the overhead of sifting through historical data. - Efficient Data Management: By updating the state rather than appending new entries for each poll, the
modem_state_index
remains lean and focused, avoiding the bloat that can come from storing redundant data. - Separation of Concerns: While the
modem_state_index
gives us a quick snapshot of the network, deep time series analytics are handled by a separate index, themodem_metrics_index
. This separation allows each index to be optimized for its specific use case—fast, real-time views in one, and detailed, historical analysis in the other. - Modem Lifetime: By keying the modems on this sha256 key we can also track the lifetime of a modem as a new entry will be created when the modem changes addresses or users. By searching on mac address we can find every address and user where a single modem has ever lived.
This design choice is what enables the system to provide both a high-level, immediate view of the network’s current state and the ability to dive deep into performance trends over time. By keeping the modem_state_index
focused and efficient, we ensure that our maps and dashboards are always responsive and up-to-date, providing the actionable insights needed to manage the network effectively.
Since We’re Here, Let’s Talk About the modem_metrics_index
While the modem_state_index
provides a fast, real-time snapshot of each modem's current status, the modem_metrics_index
is where the heavy lifting happens in terms of detailed, historical analysis. This index is designed to handle the large volume of data generated by continuously polling thousands of modems, storing it in a way that allows for deep insights into network performance over time.
The Scale of Data Collection
Let’s break down the numbers to understand the scale of what we’re dealing with:
- Polling Frequency: We poll each modem every 10 minutes.
- Number of Modems: There are 2,300 modems in the network.
- Number of Interfaces per Modem: On average, each modem has about 25 interfaces.
- Number of Metrics per Interface: We collect approximately 10 metrics per interface.
Here’s the math:
- Polls per Day: There are 144 polling intervals in a day (24 hours x 6 polls per hour).
- Documents Created per Poll: Each interface gets its own document in the index, so for 2,300 modems with 25 interfaces each, that’s 57,500 documents per polling interval (2,300 modems x 25 interfaces).
- Total Documents per Day: Multiply this by the number of polls per day:
- 57,500 documents per poll x 144 polls per day = 8,280,000 documents per day.
This gives us a ballpark figure of around 8.3 million documents being generated and stored in the modem_metrics_index
every day. This is a considerable volume of data, but the granularity it provides is unbeatable.
Indexing Strategy
To manage this massive influx of data efficiently, the modem_metrics_index
is structured as single-shard daily indices. Each day, a new index is created to store that day's modem data. This is accomplished by using a basic strategy in the poller: the base name modem_metrics_index
is combined with the current date in YYYY-MM-dd
format, appending it to the index name on every ingest. This approach helps in managing and querying data more effectively, keeping each index focused and optimized for daily queries. It also allows for easier archival or deletion of old data without affecting current operations.
In contrast, the modem_state_index
is a single, continuous index that only ever holds the latest snapshot of the modems in the network. Regardless of how frequently we poll them, this index always reflects the current state of the modems, with no accumulation of historical data. As mentioned earlier, the modem_state_index
only updates the existing records, ensuring it remains lean and fast, holding just the number of modems in the network.
Why Not Nest the Data?
One might ask, why not nest the data within each document? The reason is that while nesting might seem like a way to reduce the number of documents, it doesn’t play well with the kinds of queries we need to perform in Grafana. Nesting can complicate queries and slow down performance, especially when we’re dealing with large volumes of time series data like this. By storing each interface’s metrics in its own document, we ensure that the data remains easily accessible, queryable, and efficient to work with.
The Benefits of Granularity
While 8.3 million documents per day might sound like a lot, the level of detail this provides is invaluable. With this granularity, we can track the performance of every interface on every modem with pinpoint accuracy. It allows us to:
- Identify Trends: By analyzing the data over time, we can spot emerging issues before they become critical.
- Pinpoint Issues: If there’s a problem with a specific interface, the detailed data in the
modem_metrics_index
allows us to drill down and see exactly what’s happening, when, and where. - Optimize the Network: This level of detail helps us fine-tune the network, ensuring that we’re delivering the best possible service to every user.
In essence, while the data volume is significant, the insights we gain from this detailed, time-series data make it all worthwhile. The modem_metrics_index
is the backbone of our in-depth analysis, providing the raw data needed to maintain, optimize, and troubleshoot the network effectively.
BootstrapModemPoller: The Start of the Good Stuff
Now we’re diving into the real meat of the project—where the action begins. The BootstrapModemPoller
function is where we kick off the modem polling process, and it’s packed with several crucial steps. To keep things organized, we’ll break this down into a few sections, starting with the initial SNMP walk.
Step 1: SNMP Walk to Gather Cable Modem MAC Addresses
The very first thing BootstrapModemPoller
does is perform an SNMP walk of the CMTS to retrieve the cable modem MAC table. This step is essential because it gives us a list of all the MAC addresses associated with the CMTS, which are the unique identifiers for each modem on the network.
Here’s how it works:
- SNMP Walk of the Defined CMTS: The function starts by sending an SNMP walk request to the CMTS defined in the
.env
file passed to the-config
option when you start the program. This-config
file is mandatory; the program will not start without it. There is no default configuration because this tool is designed to run as a standalone application for any CMTS, anywhere. This design allows the tool to be flexible and adaptable, capable of running on individual cable modem networks as needed. Even if you manage multiple CMTSs, which we do, each one gets its own dedicated poller. - Loop Through Data: Once the data is returned, the function loops through the list of MAC addresses. During this loop, it processes each MAC address to prepare it for the next steps in the polling process.
- Create a Service ID to MAC Address Map: As it loops through the data, the function creates a map (or dictionary) where each entry uses the Service ID of the cable modem as the key, pointing to the corresponding MAC address. This map is crucial because it allows us to quickly reference which MAC address corresponds to which Service ID throughout the rest of the polling process.
This mapping is the foundation that enables the entire polling system to function efficiently. By organizing the modems by Service ID and associating them with their MAC addresses, we create a structure that allows for rapid querying and data retrieval, setting the stage for the detailed polling that follows.
Step 2: SNMP Walk to Gather Cable Modem IP Addresses
After the initial gathering of MAC addresses, the next critical step that BootstrapModemPoller
performs is an SNMP walk of the cable modem IP address table. This step is equally important because it allows us to associate each modem’s IP address with its corresponding Service ID and MAC address, building a more complete picture of each device on the network.
Here’s how it works:
- SNMP Walk of the IP Address Table: The function initiates an SNMP walk targeting the IP address table of the CMTS, which, like the MAC address table, is defined in the
.env
file passed via the-config
option when the program starts. This walk retrieves the list of IP addresses for all the modems connected to the CMTS. - Loop Through the Data: Once the data is collected, the function loops through each entry in the IP address table. Each entry includes both the IP address and the Service ID of the modem, which allows us to match it with the previously gathered MAC address.
- Extract the IP Address: During the loop, the function splits the value to separate the IP address from the Service ID (SSID). This ensures that the IP address is isolated and ready to be associated with the correct modem.
- Map the IP Address Using the Service ID: Using the Service ID as the key, the function places the IP address into the same map (or dictionary) that already contains the MAC address. This way, each Service ID in the map now points to both the MAC address and the IP address of the modem.
By combining both the MAC and IP addresses into the same map based on the Service ID, we create a comprehensive reference for each modem. This enriched map is critical for the subsequent steps of the polling process, ensuring that every piece of data we collect is accurately tied to the correct modem.
Here’s Where We Get Into the Good Stuff: Attaching the Node Name with a 3-Step Process
Now we’re diving into one of the most crucial parts of the BootstrapModemPoller
process: attaching the ifAlias
, which in our network is the name of the node where the modem resides. This step is a bit more involved, but it’s essential for enabling deep insights and precise filtering in Grafana. The process unfolds in three main steps, and while it’s a bit painful due to the multiple SNMP walks required, it’s worth it for the level of detail we achieve.
Step 1: Walking the Cable Bundle Table
The first step involves walking the cable bundle table. This table gives us the specific cable bundle that each modem is connected to. Here’s how it works:
- SNMP Walk of the Cable Bundle Table: The function sends an SNMP walk request to retrieve the cable bundle associated with each modem. This data is crucial because it identifies the physical grouping of channels that the modem uses to communicate with the network.
- Store the Cable Bundle in the Map: Once we have the cable bundle information, we store it in the existing map, using the Service ID (SID) as the key. This means that for each modem, we now have its MAC address, IP address, and the cable bundle it’s connected to.
Step 2: Walking the Interface Index Table
Next, we need to identify the primary interface associated with the cable bundle. This requires another SNMP walk, this time targeting the interface index table:
- SNMP Walk of the Interface Index Table: The function performs an SNMP walk to find the
ifIndex
corresponding to the primary interface of the cable bundle. TheifIndex
is essentially an identifier that the network uses to keep track of different interfaces. - Store the ifIndex in the Map: After retrieving the
ifIndex
, it is stored in the same map alongside the MAC address, IP address, and cable bundle. This index is critical for the final step, where we link the modem to its specific node.
Step 3: Walking the ifAlias Table
The final step is to attach the node name (ifAlias
) to the modem. This is done by performing another SNMP walk, this time on the ifAlias
table:
- SNMP Walk of the ifAlias Table: The function walks the
ifAlias
table, searching for theifIndex
we obtained in the previous step. TheifAlias
associated with thisifIndex
contains the name of the node that the modem is connected to. - Store the Node Name in the Map: Once the
ifAlias
(node name) is found, it is stored in the map under the Service ID. Now, each entry in the map contains the MAC address, IP address, cable bundle,ifIndex
, and node name of the modem.
The End Result
This 3-step process may be complex, but it’s powerful. By the end, we have a comprehensive map that not only includes the basic identification details of each modem but also links each modem to its specific node within the network. This linkage is what allows us to drill down to the node level in Grafana, using filters and maps to visualize the network in a highly granular way. The ability to track and analyze data at the node level is crucial for pinpointing issues and optimizing network performance.
A Quick Detour: Understanding the "Map"
Before we go any further, let's take a moment to clarify the term "map" that we've been referring to throughout the explanation. This map is actually the ModemData
struct we created back in main.go
. It’s the central data structure that holds all the information we've been gathering—from MAC addresses and IP addresses to cable bundles and node names. This struct is passed around to various functions as needed, serving as the core repository of the modem data we're working with.
The Role of the ModemData
Struct
The ModemData
struct is like a living, evolving record of each modem on the network. As we perform SNMP walks and gather more information, we update this map with new data points. Up to this point, we’ve been able to write to it whenever we need to because everything has been running in a single-threaded process. This makes it straightforward to add or modify entries in the map without worrying about conflicts or data integrity issues.
Here’s an example of what the ModemData
struct might look like in Go:
type ModemInfo struct { MACAddress string IPAddress string CableBundle string IfIndex int NodeName string } var ModemData = make(map[string]ModemInfo)
At this point in the process, the Service ID (SSID) is the key in the ModemData
map. Each Service ID maps to a ModemInfo
struct that contains all the relevant details about the modem, such as its MAC address, IP address, cable bundle, ifIndex
, and node name.
Preparing for Parallel Polling: Mutex Locking
However, as we dive deeper into the polling process, things start to get more complex. We’ll soon be moving into parallel polling, where multiple threads will be accessing and modifying the ModemData
struct simultaneously. This is where the concept of mutex locking comes into play.
In parallel processing, mutex (short for "mutual exclusion") locking is used to prevent multiple threads from modifying the same piece of data at the same time. Without mutex locks, you could end up with race conditions, where the outcome depends on the unpredictable order in which threads execute. This could lead to inconsistent or corrupted data within the ModemData
struct.
And here's something I learned the hard way: Without proper mutex locking, some processes might never know that they’ve finished processing, causing the entire program to hang. I ran into this issue many times when I first started working on this project. It was a frustrating experience, but it underscored the importance of getting the locking mechanism right.
By using mutex locks, we ensure that only one thread can modify the map at a time, preserving the integrity of the data and preventing the program from hanging. While it’s true that other threads do have to wait their turn when a lock is in place, if the locking and unlocking are properly structured, this happens in microseconds. To the human eye, it seems as if there’s no waiting at all. This efficiency is key to maintaining the high performance of the polling process.
What's Next?
We’ll get into the details of parallel polling and mutex locking later, but for now, it’s important to understand that this map—the ModemData
struct—is central to everything we’re doing. It’s the foundation that enables us to keep track of all the modems and their associated data, and managing it correctly is key to the success of the entire process.
We Are Almost to Modem Polling
We’ve covered a lot of ground so far, laying the foundation for the modem polling process. Let’s quickly recap what we’ve accomplished:
- Initialization in
main.go
: We set up the program by processing CLI options, loading configuration files, and verifying our connection to OpenSearch. We also created theModemData
struct, which acts as the central repository for all the modem data we’re collecting. - Gathering Data in
BootstrapModemPoller
:- We performed an SNMP walk of the CMTS to retrieve the list of MAC addresses and IP addresses for each modem, storing this information in the
ModemData
map, keyed by the Service ID (SSID). - We then carried out a more complex process to attach the
ifAlias
(node name) to each modem, allowing us to drill down to the node level in our network analysis.
- We performed an SNMP walk of the CMTS to retrieve the list of MAC addresses and IP addresses for each modem, storing this information in the
- Understanding the Role of the
ModemData
Map: We discussed how theModemData
struct holds all the collected information and the importance of using mutex locking to ensure data integrity as we move into parallel polling.
Now, we’re almost ready to start the actual modem polling. But before we dive into that, there’s one more critical step: converting the ModemData
map to be keyed by MAC address. Up until now, we’ve been using the Service ID as the key because it was the most logical identifier during the data collection phase. However, as we move forward with modem polling, the MAC address becomes the most important identifier.
Why the Change?
From this point on, the Service ID (SSID) is no longer relevant to the tasks we need to perform. Instead, we’ll be updating and interacting with the ModemData
map based on each modem’s MAC address. This shift is necessary because all subsequent operations, including the SNMP queries and data updates, are keyed off the MAC address rather than the Service ID.
Converting the map to be keyed by MAC address is straightforward, but it’s an essential step that ensures the rest of the process runs smoothly. Once this conversion is complete, we’ll be fully prepared to begin the modem polling phase.
Example to Convert ModemData
to be Keyed by MAC Address
package main import "fmt" // ModemInfo holds details about each modem. type ModemInfo struct { MACAddress string IPAddress string CableBundle string IfIndex int NodeName string } // Example ModemData map keyed by Service ID. var ModemData = map[string]ModemInfo{ "SID12345": {"00:1A:2B:3C:4D:5E", "192.168.0.10", "Bundle1", 101, "NodeA"}, "SID67890": {"00:1A:2B:3C:4D:5F", "192.168.0.11", "Bundle2", 102, "NodeB"}, } func main() { // Loop through the original ModemData map. for sid, modem := range ModemData { // Use the MAC address as the new key in the same map. ModemData[modem.MACAddress] = modem // Delete the old entry keyed by Service ID. delete(ModemData, sid) } // Output the modified map to verify the conversion. fmt.Println("ModemData keyed by MAC address:") for mac, data := range ModemData { fmt.Printf("MAC: %s, IP: %s, Node: %s\n", mac, data.IPAddress, data.NodeName) } }
The Heavy Lifting: Parallel Polling with StartModemPollerThreads
Now we’re getting into the heart of the operation—the heavy lifting. This is where we pass the ModemData
struct to StartModemPollerThreads
and initiate the process of parallel polling. The approach we take here involves spinning up 200 threads, with each thread responsible for polling a single modem at a time.
Where It All Begins
This is the starting point of the parallel polling process. By launching 200 threads simultaneously, we’re able to poll a large number of modems in parallel, significantly speeding up the data collection process. Each thread works independently to gather data from its assigned modem, ensuring that the entire network can be polled in a fraction of the time it would take sequentially.
A Quick Overview of Threading
When running this many poller threads at the same time, there are a couple of key considerations to keep in mind:
- Network Socket Saturation: Spinning up 200 threads means we’re opening 200 simultaneous network connections. We have to be careful not to saturate the available network sockets on the polling host. If too many sockets are used at once, it can lead to resource exhaustion, causing delays or failures in the polling process.
- Network Load Management: Additionally, we must avoid overloading the network with poll requests. Sending out a large volume of SNMP requests at once can put significant strain on the network, especially if it’s already congested. There’s a fine balance here, and for our setup, 200 threads strike the right balance between efficiency and network load.
Scalability and Performance
The current configuration of 200 threads works well for our network, allowing us to efficiently poll 2,300 modems within a 10-minute cycle. However, this setup is also capable of scaling to handle many more modems if needed.
When the network is congested, it can take around 90 seconds to complete the poll cycle for 2,300 modems. If we spread that polling over a full 10-minute interval, we can calculate the potential capacity:
- Poll Time for 2,300 Modems: 90 seconds.
- Total Time Available per Cycle: 10 minutes = 600 seconds.
- Potential Modem Capacity: 15,341 modems.
This means that, theoretically, with 200 threads, we could handle polling over 15,000 modems in a 10-minute cycle, even under network congestion. This capacity ensures that our polling system remains robust and scalable, capable of adapting to larger networks as needed.
We’ll delve into the specifics of what happens for each modem in the following sections, breaking down the exact steps and optimizations that make this parallel polling both fast and effective.
A New Map, the InterfaceData Struct
At this point in the process, it’s practical to store the filtered interface data in a new map within the ModemData
struct, named InterfaceData
. This map serves as a temporary holding area for the interfaces that are relevant based on the criteria established earlier. While the InterfaceData
map is a convenient place to organize and manage these details for now, it’s important to note that we won’t be using this exact structure when we eventually ingest the data into our system.
Basically the struct looks like this:
type InterfaceData struct { IfIndex int IfDescr string docsIfDownChannelPower float64 docsIfSigQUnerroreds int64 docsIfSigQCorrecteds int64 docsIfSigQUncorrectables int64 docsIfSigQSignalNoise float64 docsIfSigQMicroreflections float64 docsIfCmStatusT3Timeouts int64 docsIfCmStatusT4Timeouts int64 docsIfCmStatusRangingAborteds int64 docsIfCmStatusTxPower float64 } type ModemData struct { MacAddress string Hostname string InterfaceData map[string]InterfaceData // Other fields like user info, modem type, etc. }
Polling the Modem: Filtering Interfaces with ProcessModemIfType
When the poller thread gets a modem passed to it from looping through the ModemData
map, the first task it undertakes is to poll the ifType
table. This is done using the ProcessModemIfType
function. The reason for starting with the ifType
table is that not all interfaces on a modem are relevant for long-term polling. Some modems can have a dozen or more interfaces, many of which don’t provide useful data for the purposes we care about.
Filtering by Interface Type
To make the polling process more efficient and focus on the data that truly matters, we filter out only the specific types of interfaces that are relevant. In our case, we are only interested in ifType
values 127, 128, and 129. Here’s what each of these represents:
- Type 127 (DocsCableDownstream): This represents the downstream channels on a DOCSIS cable modem. These interfaces handle the data flowing from the network to the modem, which is crucial for monitoring download speeds, signal quality, and overall network performance on the user's end.
- Type 128 (DocsCableUpstream): This represents the upstream channels on a DOCSIS cable modem. These interfaces manage the data being sent from the modem back to the network. Monitoring these interfaces is essential for ensuring that the modem can reliably send data, which is critical for services like VoIP and real-time communication.
- Type 129 (DocsCableMacLayer): This represents the MAC layer interface on the modem, which is responsible for handling the lower-level data link functions. This includes tasks like error detection and correction, as well as managing data frames on the network. While not as immediately visible to the end user as upstream or downstream performance, the MAC layer is fundamental to the modem’s ability to maintain a stable and efficient connection.
By filtering out these specific interface types, we ensure that the polling process remains focused on the metrics that are most critical for maintaining and analyzing network performance. This not only reduces the load on the polling system but also ensures that the data we collect is relevant and actionable.
Basically it is done like this:
// Loop through the ifType data for ifIndex, ifType := range ifTypeData { switch ifType { case 127: modem.IfTypes[ifIndex] = "DocsCableDownstream" case 128: modem.IfTypes[ifIndex] = "DocsCableUpstream" case 129: modem.IfTypes[ifIndex] = "DocsCableMacLayer" } }
In the next sections, we’ll dive deeper into how we poll these interfaces, gather the relevant data, and update our ModemData
map with this information. Each step is designed to be as efficient as possible while ensuring that we don’t miss any critical data points.
On To Gathering the Data
Building OID Chunks: Optimizing the Polling Process
Once we have stored the ifIndex
and ifType
of the interfaces we want to poll, the next step is to build OID (Object Identifier) chunks using the BuildOIDChunks
function. This step is crucial for optimizing the efficiency of our SNMP polling process, ensuring that we balance performance with network usage.
Why Build OID Chunks?
Polling each interface individually for every metric can be inefficient, especially when dealing with a large number of modems and interfaces. Instead, we group the OIDs into chunks and run multiple SNMP Get requests against these chunks. This approach reduces the overhead of making separate requests for each metric, making the polling process faster and more efficient.
How It Works
- Reference Data from SharedVarsAndFunctions: The
BuildOIDChunks
function references a dataset fromSharedVarsAndFunctions
that specifies which metrics we need to collect from each type of interface. These metrics are tailored to the specific needs of monitoring downstream, upstream, and MAC layer interfaces. - Chunking the OIDs: The identified OIDs are then grouped into chunks, with each chunk containing up to 25 OIDs. Through experience and testing, this chunk size has been found to offer the best balance between polling performance and network load. Larger chunks could overwhelm the network, while smaller chunks might result in inefficient use of resources.
- Calculation of Chunks: The total number of chunks generated for a modem depends on the number of interfaces we’re monitoring and the number of metrics per interface. For example:
- If a modem has 25 relevant interfaces and we’re collecting 10 metrics per interface, this would result in 25×10=250 OIDs. With a chunk size of 25, this modem would end up with 10 chunks.
Side Note: Why We Don’t SNMP Walk the Entire Modem or Tables
You might wonder why we don’t simply SNMP walk the entire modem or all the available tables to gather data. After all, wouldn’t that give us everything in one go? While that’s true, it’s not the most efficient approach, and efficiency is key in a process like this.
The Problem with Full SNMP Walks
I’ve tested this approach extensively, and the results are clear: performing a full SNMP walk of an entire modem or its tables takes about four times longer than collecting only the data we actually need. Why? Because full SNMP walks retrieve every single piece of information, including metrics for interfaces that aren’t relevant to our monitoring goals along with data that we do not need for monitoring. This means we end up sifting through a lot of unnecessary data, wasting both time and network resources.
Focused Data Collection
Our approach is geared towards efficiency. By chunking the OIDs and targeting only the specific interfaces and metrics we care about, we dramatically reduce the amount of data we have to process. This method ensures that we get exactly what we need—nothing more, nothing less—allowing the polling process to run as quickly and smoothly as possible.
In essence, the goal here isn’t to get everything; it’s to get what’s important. This focused approach allows us to maintain high performance and keep the network load manageable, which is especially critical when dealing with large-scale deployments.
Next Steps
After building these OID chunks, the poller threads can execute SNMP Get requests more efficiently. Each thread will process its assigned chunks, retrieving multiple metrics in a single request, which minimizes the total number of SNMP operations required. This efficiency is critical when polling large networks, where time and resource management are key.
In the upcoming sections, we’ll explore how these SNMP Get requests are executed against the OID chunks and how the collected data is processed and integrated back into the ModemData
struct.
And Finally Here We Are: Collecting the Data We Actually Want with GetSNMPChunks
After all the groundwork we’ve laid—filtering interfaces, building OID chunks, and ensuring everything is set up for efficient polling—we finally arrive at the core of our operation: collecting the actual data with GetSNMPChunks.
The Role of GetSNMPChunks
The GetSNMPChunks function is where we execute the SNMP Get requests for the OID chunks we’ve carefully constructed. This is the step where all the preparation pays off, as we efficiently gather the metrics that matter most to our network monitoring.
We've been using Go routines since we started gathering the ifType, which is where Go routines really excel compared to forking multiple processes in Perl. This poller runs on a VM that also hosts Logstash, Grafana, and OpenSearch Dashboards. Despite the limited resources—8 processor cores and 4GB of RAM—the poller operates efficiently, rarely exceeding a load of 1 and using only about 100MB of memory during the polling process. All of my testing when trying to optimize Perl to handle this many modems in parallel would easily drive the system load to 40. But let's also remember, the tech and stack were much different then.
How It Works
- Processing Each Chunk: The function loops through the OID chunks generated for each modem. Since we’ve already optimized these chunks to contain only the relevant OIDs, each request is streamlined to fetch precisely what we need.
- Sending SNMP Get Requests: For each chunk, an SNMP Get request is sent to the modem. This request retrieves the specified metrics for the selected interfaces, such as signal strength, noise levels, error counts, and more.
- Handling Responses: The responses from these SNMP Get requests are parsed and processed. The collected data is then integrated back into the ModemData struct, updating the modem’s current state with the latest metrics.
- Efficiency in Action: Thanks to the chunking strategy, the GetSNMPChunks function is able to retrieve data from multiple interfaces in a single request. This approach drastically reduces the number of SNMP operations required, minimizing both the time taken and the network load generated by the polling process.
Making it Human Readable
One key aspect that makes our data processing more effective is converting the numerical OIDs into their text representations using the ReverseOID map. This step is crucial for making the data more accessible and understandable. By translating these long strings of numbers into meaningful labels, we simplify the process of building analytics around them, allowing us to focus on insights rather than memorizing complex OID sequences.
The Importance of Targeted Data Collection
At this stage, it becomes clear why we went through the trouble of filtering interfaces and building OID chunks. By focusing our efforts on the specific data we actually want, we avoid the pitfalls of inefficiency that come with full SNMP walks. Instead, GetSNMPChunks allows us to quickly and effectively gather the most critical metrics, ensuring that our monitoring system is both fast and responsive.
Enriching The Data
Getting the User Info From Parse Dashboard
In this phase, we have finished the threaded polling and are back in single-thread mode. Now we retrieve user information from the Parse Dashboard by executing a query via the Parse API. The returned data is in JSON format and includes essential details such as username, address, revenue area, modem type, modem serial, and more.
The MAC addresses in Parse Dashboard are stored in a no-colon, uppercase format. As we loop through the JSON output, we create a dictionary (map) where each entry is keyed by these formatted MAC addresses. This dictionary will be crucial in the next step, where we enrich the ModemData
struct by matching these MAC addresses to the corresponding modem data.
This process is managed by the QueryUserInfoFromParser
function, which ensures that all user information is efficiently organized and ready for integration in the following data enrichment phase.
Final Enrichment
In the final stage of data preparation, we pass both ModemData
and UserInfo
to the EnrichModemData
function. This function is responsible for looping through each entry in ModemData
and performing the crucial task of matching and merging user information.
As the function iterates through ModemData
, it first converts each MAC address to the no-colon, uppercase format to align with the format used in our UserInfo
data. It then searches for a corresponding entry in the UserInfo
map.
When a match is found, the relevant user information—such as username, address, revenue area, modem type, and serial number—is merged into the ModemData
struct at the original MAC address. This enriched dataset now contains both technical metrics and user-specific details.
If there is no match found for a user it is marked as "No User Found" in all strings of the meta data.
This level of enrichment is vital as it allows us to dive deep into the data, enabling street-level analysis and visualization. Whether troubleshooting or optimizing the network, this detailed information ensures that we have a comprehensive view of each modem's context within the network.
On To the Ingestion
With all the necessary data now consolidated in ModemData
, the next step is to ingest this enriched information into OpenSearch. The IngestModemDataToOpenSearch
function is responsible for this process, where we pass in the ModemData
that now contains both the technical metrics and user-specific details.
During the polling process, a key called poll_status
was set for each modem. If the modem was polled successfully, this status is marked as "Success." However, if any errors occurred during polling, these errors are captured and recorded. If any polling attempts were missed, the reason for the failure is noted in a field called poll_message
, and the poll_status
is set to PollFailed. If any values were detected as being outside normal operating range this key is set to OutOfBounds. And a message is created for poll_message stating which values were out of bounds. This status management was handled in the PollChunks
portion of the program.
As we begin the ingestion process, the modem’s state data, including all user information and the poll_status
, is stored separately in the modem_state
index. This forms the foundation of our data, capturing the overall status and metadata for each modem.
However, the bulk of our data—and where the millions of documents land—comes from the interface data for each modem. For every modem, we loop through its InterfaceData
, and each interface, identified by ifIndex
and ifDescr
, is stored as an individual document. These documents include all the relevant metrics for that interface, such as signal quality and error counts, as well as key pieces of user-specific information like the MAC address, username, and the SHA-256 hash key we created earlier to serve as the _id
in the modem_state
index.
Using the SHA-256 hash as the _id
in the modem_state
index and as sha_id_key
within each interface document allows us to efficiently tie user metadata—including coordinates, serial numbers, and all other collected information—to each interface. This method avoids the need to store all pieces of metadata redundantly for every interface, which would otherwise double the size of the index.
Finally, by leveraging Grafana dashboard variables, we can seamlessly integrate all of this data into a cohesive dashboard. The well-designed visuals can tie everything together based on the SHA-256 key, providing a powerful and efficient way to analyze and visualize the data.
Ans That Is How We Poll 2,300 Modems In 30 Seconds
So there you have it, 5.5 hours and lots of edits later —what started as a seemingly simple task of polling 2,300 modems in 30 seconds has turned into an epic journey through the world of network monitoring, data enrichment, and efficient data ingestion. We’ve gone from understanding the basics of a cable modem to deep-diving into the intricacies of Go routines, SNMP polling, and even hashing strategies to keep our data storage lean and mean.
Along the way, we’ve explored how to transform raw, unmanageable data into something both powerful and actionable, making it possible to drill down to the street level and visualize the health of our network in near real-time. It’s been a long road, and somewhere between discussing interface filtering and bulk ingestion, what was supposed to be a short blog post evolved into what might as well be a technical novel.
For that, I suppose I owe you an apology—or perhaps a congratulations, if you’ve made it this far! While it might not have been the quick read I initially intended, I hope this deep dive has been as enlightening for you as it has been for me in putting it together.
After all, when you’re working in tech, sometimes the “simple” things are anything but. They’re complex, fascinating, and yes, a bit lengthy to explain—but that’s part of what makes this work so rewarding.
Thanks for sticking with me through this deep exploration. Whether you’re here for the details or just the bigger picture, I hope you’ve found something valuable to take away. And with that, I officially wrap up this extensive overview on how we manage to poll 2,300 modems in just 30 seconds. Until the next “short” blog post… cheers!
-I Think That's It
--Bryan Vest